Home > Modernizing USDA Livestock Grading with Machine Vision and LiDAR
Modernizing USDA Livestock Grading with Machine Vision and LiDAR
CLIENT
USDA
INDUSTRY
- Agriculture
01
CASE
Modernizing USDA Livestock Grading with Machine Vision and LiDAR
02
Overview
Project Synopsis: Ensemble is supporting the design and development of a USDA-sponsored proof of concept (POC) to capture non-contact visual measurements of livestock using advanced and novel technologies. USDA’s Agricultural Marketing Service (AMS) livestock grading subject matter experts and methodologies were leveraged along with tools such as LiDAR cameras to create skeletal mappings using artificial intelligence and machine vision algorithms.
The resulting technology platform proved feasible and has the potential to enhance AMS reporter’s toolset and modernizing grading methods while empowering farmers and producers with real-time insights for market participation, purchasing, and herd evaluation.
Background of the Problem: The USDA AMS team is exploring innovative tools to enhance current livestock evaluation methods. Visual contactless measurements powered by emerging technologies can help automate data capture, reduce human error, improve data-driven decision-making, and scale AMS reporting coverage. Ensemble developed technical and operational paths for web application development, aligning with USDA grading standards and supporting scalability and field usability.
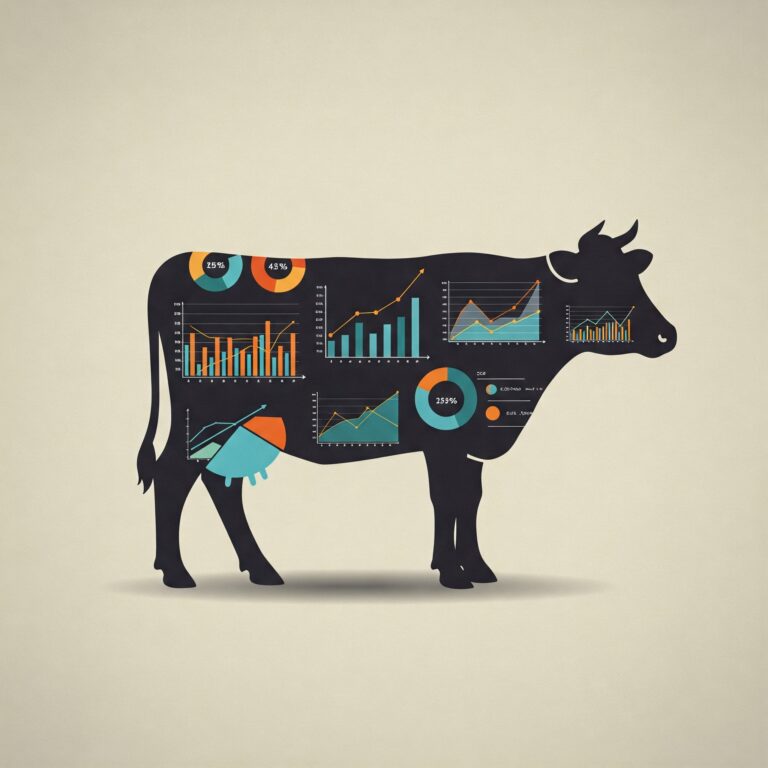
03
OUR
Approach
Ensemble has extensive experience building AI/ML-driven platforms, web/mobile applications, and automation tools to improve data collection, accuracy, and decision-making. Our technical solution integrates machine learning, computer vision, and sensor-based measurements within a modular architecture to enable fast and accurate scoring.
- Requirement Analysis- Worked with USDA AMS and stakeholders to identify key livestock attributes and capture technical and functional needs through workshops.
- Data Collection- The team collected real-world livestock data via several in-person data capture sessions. We conducted exploratory analysis and built labeled datasets supported by robust data cleaning and metadata augmentation.
- Algorithm and Model Development- Created computer vision algorithms and machine learning models to enable feature selection and build out 2D-to-3D body mapping and recognition, with LiDAR integration for enhanced accuracy
- Prototyping- A modular cloud native application was built to demonstrate data processing and scoring capabilities using multimedia datasets.
05
Results
At the conclusion of the application development, the following results were delivered:
- Roadmap for enhanced efficiency in livestock grading through automation
- Improved data accuracy and reliability for USDA AMS reporting
- Data-driven decisions support for cattle grading
- Adoption strategy for applications beyond feedlot settings and across species
This proof of concept marks a step toward faster, smarter, and more reliable livestock grading, laying the groundwork for technological advancements that will strengthen U.S. agriculture.